In a recent preprint study published on Preprints with The Lancet*a team of researchers evaluated the use of prediction models together with clinical information, metatranscriptomics and the lower respiratory tract microbiome.
Their findings suggest that machine learning models can become a rapid diagnostic tool, bypassing the morbidity and mortality associated with conventional microbiological testing.
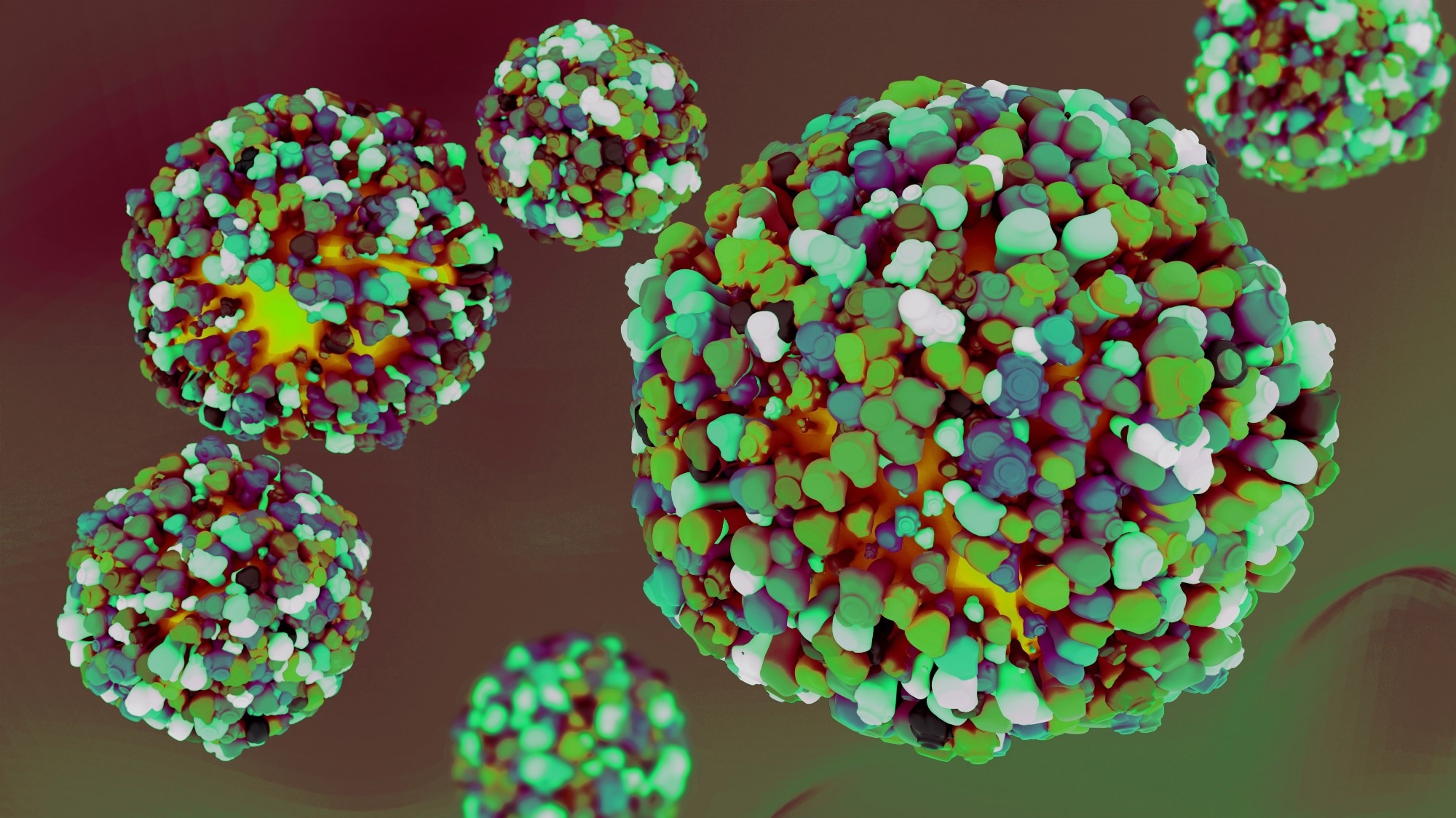
Study: Integrating the respiratory microbiome and host immune response using machine learning for the diagnosis of lower respiratory tract infections. Image Credit: MZinchenko/Shutterstock.com
*Important Notice: SSRN publishes preliminary scientific reports that are not peer-reviewed and, therefore, should not be considered conclusive, guide clinical practice/health-related behavior, or treated as established information.
LRTI diagnosis
Lower respiratory tract infections (LRTIs) are responsible for more than 3 million deaths annually, making them one of the leading infectious causes of human mortality globally. The high morbidity and mortality of LRTIs has historically been attributed to the conventional diagnosis of respiratory infection. Traditional diagnosis lacks sensitivity, fails to identify 60-70% of causative agents, and requires 24-48 hours or more for infection to be characterized.
LRTIs are known to exhibit an extreme variety and variability in their symptomatic presentation, many of which overlap with non-infectious conditions such as asthma, chronic obstructive pulmonary disease (COPD), or cystic fibrosis. Doctors therefore prefer to delay diagnosing a patient or risk misdiagnosing the disease, which could be life-threatening.
Recent studies challenge the classical view of the pathogenesis of LRTI: traditional knowledge assumes that the lungs are initially sterile. It takes a critical volume of pathogenic microbes invading the lungs to overwhelm the immune response, resulting in rapid infection.
A growing body of research using microbial genomes proposes that LRTIs arise due to a combination of low microbial species diversity, high overall biomass, and host inflammatory response.
Alternations in respiratory tract microbiomes have also been observed in non-infectious diseases such as asthma, marking microbiome studies as critical in the identification and characterization of LRTI. An emerging field, next-generation metagenomic sequencing (mNGS) is being tested as a viable, rapid, and sensitive alternative to traditional diagnostic tools.
mNGS requires microliter volumes of patient samples and could provide accurate diagnoses in minutes to hours versus days conventional diagnostic tools currently take.
About the study
In the present preprint study, researchers attempted to collect and combine the respiratory microbiome and transcriptional profile of the host with clinical data. They then trained a machine learning model and tested its speed and diagnostic accuracy when fed the collected data.
The researchers began by enrolling patients suspected of having LRTI at Peking University People’s Hospital, Beijing, between May 2020 and January 2021. After screening for radiography, clinical presentation, and demographics in line with the US Centers for Disease Control/National Healthcare Safety Network (CDC/NHSN), 136 participants were selected for the study.
All participants received traditional microbiological and serological tests for the diagnosis of LRTI. Researchers also collected bronchoalveolar lavage fluid (BALF) for characterization and model training. BALF was sequenced for both DNA and RNA. The RNA reads were screened against the human transcriptome and SILVA rRNA database to ensure that the remaining reads belonged to the lung microbiome.
The transcriptome and host microbiome were correlated and standardized by comparing the expression of transcripts per million (TMP) in hosts with the relative concentration of microbial flora. This data was then used to train machine learning models.
Researchers examined 11 identifying variables from clinical markers, microbial flora abundance, and host TMP upregulation. Random forest models were used, using data from 91 participants for algorithm training and 45 for testing.
Study results
Of the 136 patients enrolled in the study, 81 were found to have LRTI and formed the LRTI cohort, while the remaining 55 were enrolled in the non-LRTI cohort. LRTI-positive individuals were found to have a significantly greater amount of prior antibiotic use than their non-LRTI counterparts.
Notably, laboratory findings, including white blood cell (WBC) counts and markers of inflammation, did not differ between the two groups. This highlights the low characterization power of conventional diagnostic tools.
Patients with LRTI had significantly reduced BALF microbiota diversity compared to non-LRTI samples. Relative microbiota abundance was also different between groups, with BALF of LRTI samples representing the high abundance of pathogens Klebsiella pneumoniae, Stenotrophomonas maltophilia, Pseudomonas aeruginosa, AND Streptococcus pneumoniae.
In contrast, BALF from the non-LRTI group showed the highest abundance of Halomonas pacifica, a symbiont normally found in healthy lungs and airways. Pathogenic microbes in the LRTI samples were either absent or found in trace amounts in the non-LRTI group.
Transcriptome analyzes revealed 674 differentially expressed genes (DEGs). Of these, BALF from the LRTI cohort revealed that 613 DEGs were upregulated, while the remaining 61 were downregulated compared with the non-LRTI cohort. Screening against the Kyoto Encyclopedia of Genes and Genomes (KEGG) revealed that DEGs upregulated by LRTI were associated with pathogen infection pathways.
Correlations between microbiota diversity and host transcriptomes suggest that 31 host genes (and their expression levels) are associated and vary according to the ratio of normal LRT flora to pathogenic microbes.
Training the random forest model using this data predicted LRTIs using 70 characteristics (11 clinical, 39 lung microbiome, 20 host response). The diagnostic accuracy of the model was found to be 88.2% and results were obtainable within hours, both significant improvements over traditional diagnostic approaches.
The fundamental limitations of this study are that mNGS is currently extremely expensive and requires high technical requirements. Furthermore, while these machine learning models could serve as diagnostic markers of LRTI, they in no way characterize or explain the biological pathways or functions of the observed microbiota-host transcriptome interactions.
Conclusions
This pre-print study represents a new approach to the diagnosis of lower respiratory tract infection. Traditionally, diagnoses of LRTI can take several days and describe low sensitivity to more than 60% of infectious agents. These aspects result in misidentification of the disease and delays in intervention, significantly contributing to morbidity and mortality.
In this study, researchers characterized microbial abundance in the LRTI and non-LRTI cohorts, which they associated with host transcriptome and response data. This data was used to train machine learning models, which were subsequently able to correctly diagnose 88.2% of LRTI patients in a fraction of the time taken by conventional techniques.
This research, if validated during peer review and developed to reduce its intrinsic high cost, could help clinicians diagnose LRTI quickly and accurately in the future, thereby reducing the high mortality associated with the disease.
*Important Notice: SSRN publishes preliminary scientific reports that are not peer-reviewed and, therefore, should not be considered conclusive, guide clinical practice/health-related behavior, or treated as established information.
Magazine reference:
- Preliminary scientific report. Chen H, Qi T, Guo S, et al. (2023). Integrating the respiratory microbiome and host immune response using machine learning for the diagnosis of lower respiratory tract infections. Preprints with The Lancet. doi: 10.2139/ssrn.4505343 https://ssrn.com/abstract=4505343
#potential #machine #learning #rapid #diagnosis #LRTI
Image Source : www.news-medical.net