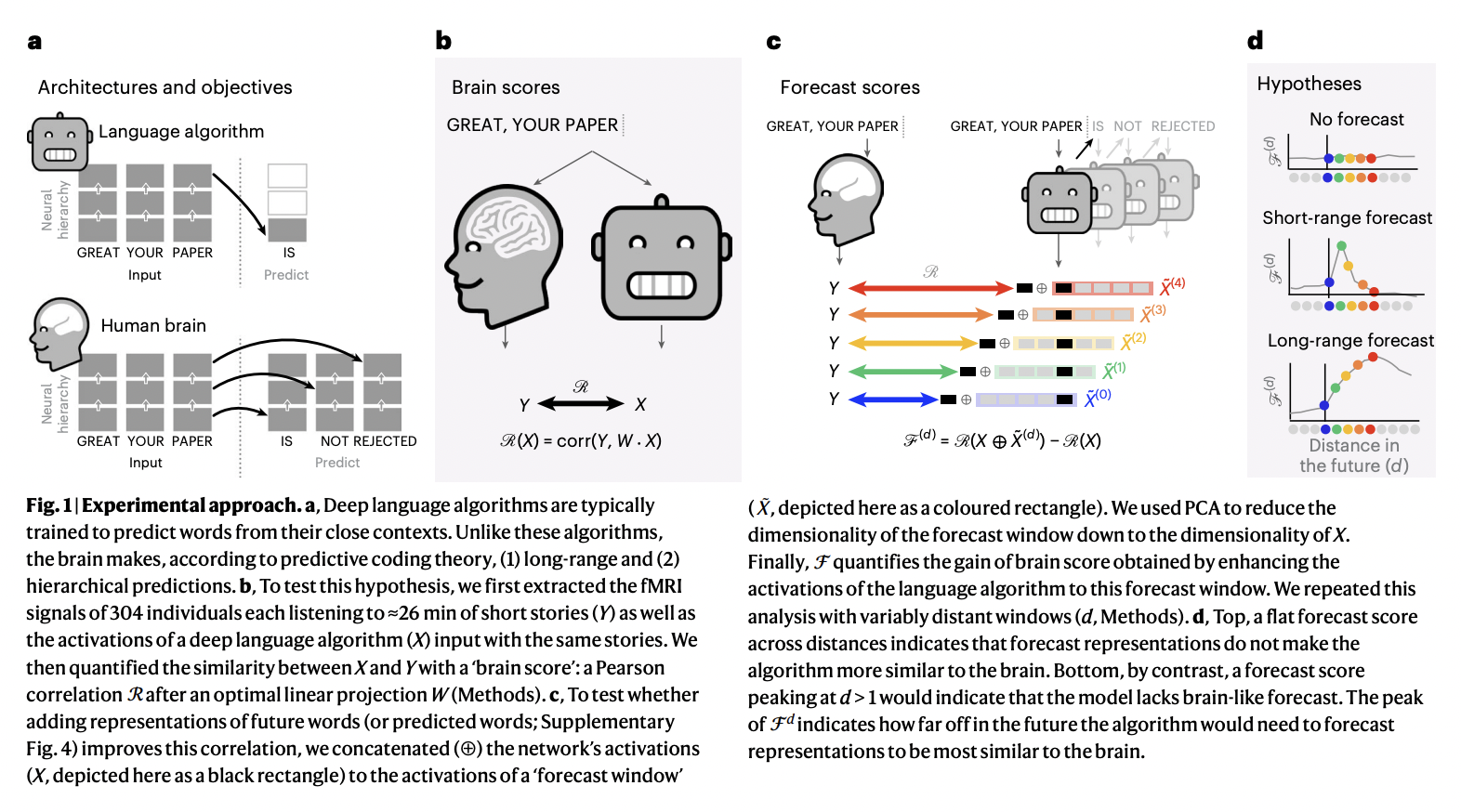
Deep learning has made great strides in text generation, translation, and completion in recent years. Algorithms trained to predict words from their surrounding context have been instrumental in achieving these advances. However, despite access to large amounts of training data, deep language models still need help performing tasks such as long story generation, summarization, coherent dialogue, and information retrieval. It has been shown that these models need help capturing syntax and semantic properties, and their linguistic understanding must be more superficial. Predictive coding theory suggests that a human’s brain makes predictions at multiple time scales and levels of representation through the cortical hierarchy. Although studies have previously shown evidence of speech predictions in the brain, the nature of the predicted representations and their temporal scope remain largely unknown. Recently, researchers analyzed the brain signals of 304 people listening to short stories and found that enhancing deep language models with long-range, multilevel predictions improved brain mapping.
The results of this study revealed a hierarchical organization of language predictions in the cortex. These findings are in line with predictive coding theory, which suggests that the brain makes predictions across multiple levels and timescales of expression. Researchers can bridge the gap between human language processing and deep learning algorithms by incorporating these ideas into deep language models.
The present study evaluated specific hypotheses of predictive coding theory by examining whether cortical hierarchy predicts different levels of representations, spanning multiple time scales, beyond the neighborhood and word-level predictions usually learned in deep language algorithms. The modern deep language patterns and brain activity of 304 people listening to spoken stories were compared. Activations of deep language algorithms integrated with high-level and long-range predictions were found to better describe brain activity.
The study made three main contributions. Initially, the supramarginal gyrus and the lateral, dorsolateral, and inferior frontal cortices were found to have the greatest prediction distances and actively anticipate future linguistic representations. The superior temporal sulcus and gyrus are best modeled by low-level predictions, while high-level predictions better model the mid-temporal, parietal, and frontal regions. Second, the depth of predictive representations varies along a similar anatomical architecture. Ultimately, semantic traits, rather than syntactic ones, were shown to be the ones that influence long-term predictions.
According to the data, the lateral, dorsolateral, inferior, and supramarginal gyri have been shown to have the longest predicted distances. These cortical areas are related to high-level executive activities such as abstract thinking, long-term planning, attention regulation, and high-level semantics. According to the research, these regions, which are at the top of the linguistic hierarchy, can actively anticipate future linguistic representations in addition to passively processing past stimuli.
The study also demonstrated variations in the depth of predictive representations along the same anatomical organization. The superior temporal sulcus and gyrus are best modeled by low-level predictions, while high-level predictions better model the mid-temporal, parietal, and frontal regions. The results are consistent with the hypothesis. In contrast to today’s linguistic algorithms, the brain predicts representations at different levels rather than just those at the word level.
Ultimately, the researchers separated brain activations into syntactic and semantic representations, finding that semantic rather than syntactic characteristics influence long-term predictions. This finding supports the hypothesis that the heart of long-form language processing may involve high-level semantic prediction.
The overall conclusion of the study is that benchmarks for natural language processing could be improved and models could become more brain-like by consistently training algorithms to predict numerous timelines and levels of representation.
Check out thePaper, DatasetsANDCode.All the credit for this research goes to the researchers of this project. Also, don’t forget to subscribeour 15k+ ML SubReddit,Discord channel,ANDEmail newsletterwhere we share the latest news on AI research, cool AI projects and more.
Niharika is a technical consulting intern at Marktechpost. She is a third year student, currently pursuing her B.Tech at Indian Institute of Technology (IIT), Kharagpur. She is a very enthusiastic individual with a keen interest in machine learning, data science and artificial intelligence and an avid reader of the latest developments in these fields.
#Deep #language #models #learning #predict #word #context #human #brain
Image Source : www.marktechpost.com